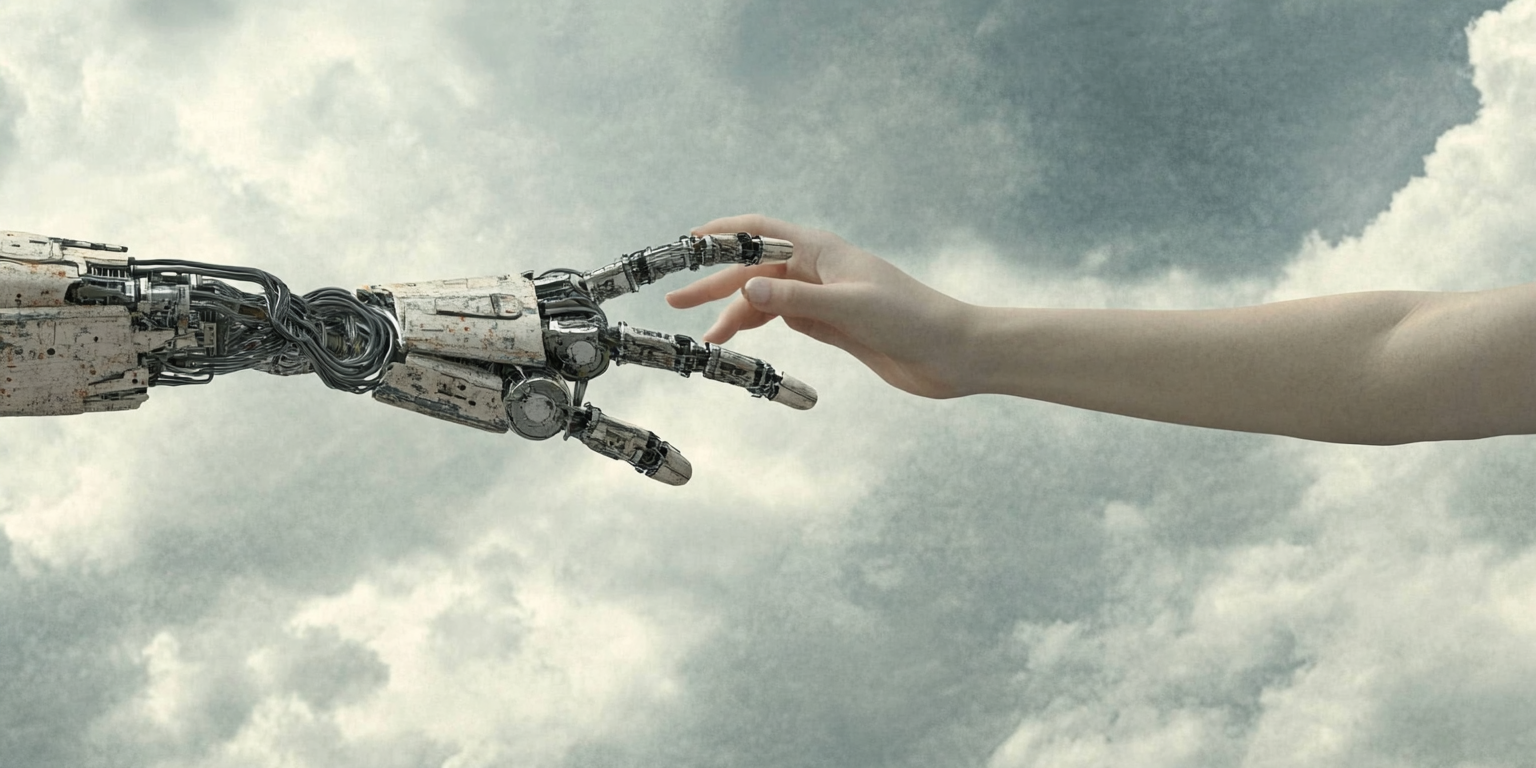
Why do so many AI initiatives fail?
AI is a hot topic on which many people find themselves lost. It’s hardly a surprise - we are dealing with a new, fundamentally different kind of technology.
The game has changed. Existing strategies and tools at our disposal simply don’t apply anymore. This is, in my opinion, the main reason why about half AI initiatives fail 1. Let me explain…
Let’s start with what I will call “Traditional Digital Transformation”. While it was never truly meant to be this way, many (but not all) digital transformation initiatives end up focusing on how to introduce new digital tools to improve efficiency and then mistakenly identify efficiency for productivity. You may disagree with this view, and that’s fair, but this is what I’ve observed so far through 100s of conversations with clients and competitors. If you give someone Microsoft Word they will likely be more efficient at writing text, but there is absolutely no guarantee that the text they produce will be any more valuable if they didn’t have this new digital tool. Productivity still remains solely on the side of the human.
Viewing AI through the lens of Traditional Digital Transformation is a lost opportunity at best. AI is not just another digital tool, it is an assistant. We won’t use it, we will work with it. In addition, digital tools always require humans to adapt to them. With AI, we have an opportunity to have technology truly adapt to us instead of the other way around. It is foolish to let that opportunity slip away.
Some people are trying to view AI through the lens of workflow and process automation. There are good reasons processes are so popular: they allow you to operationalise efficiency and ensure consistency across the organisation. McDonalds, for example, is a very process driven company and it is their processes that ensure that you can eat the same Big Mac in Japan and Belgium. Similarly, Toyota pioneered a set of legendary lean manufacturing processes which made it into the automotive powerhouse it is today.
AI typically enters into processes as a way to make individual process steps more efficient. Sounds reasonable, except that there is one common practical problem: past digital technologies have already automated most business processes as far as it was possible. Adding AI into the mix may result in marginal improvements.
The other reason why AI for process automation often fails is that it doesn’t alleviate the core issues with the process. For example, think of the way large insurance companies may process insurance inquiries and claims. If you want a process that ensures you’ll be able to handle every type of claim/inquiry, good luck with that. The complexity of inputs and outputs you have to handle is so vast that it would inevitably lead to an overly complicated process machinery which is hard to maintain, difficult to onboard people on and at the end of the day may not reach the efficiency objectives you desire. There is no amount of AI you can sprinkle on top of the process that will make it work because here AI is not addressing the core issues: rigidity and lack of flexibility in the process driven methodology.
A better way to handle objectives with complex inputs/outputs is to turn to objective driven setups, where you don’t focus on procedures, tasks and processes but on skills necessary to meet an objective. In other words, people with particular skills collaborate to reach a common objective. This approach has the advantage that it’s flexible, able to handle complexity and is more resilient than the typical process (in the context of intellectual work). However, it suffers from the fact that it’s more difficult to maintain consistency and predict outcomes (after all, we’re only human).
Yesterday’s digital technology was not sophisticated enough to be helpful as a part of a team - there was no true complement to human intelligence. But this has changed in recent years, and we are on the brink of unlocking unprecedented opportunities for productivity and efficiency with AI. For the first time, we have the opportunity to expand the scope of technology to concepts such as teams and roles of individuals in an organisation.
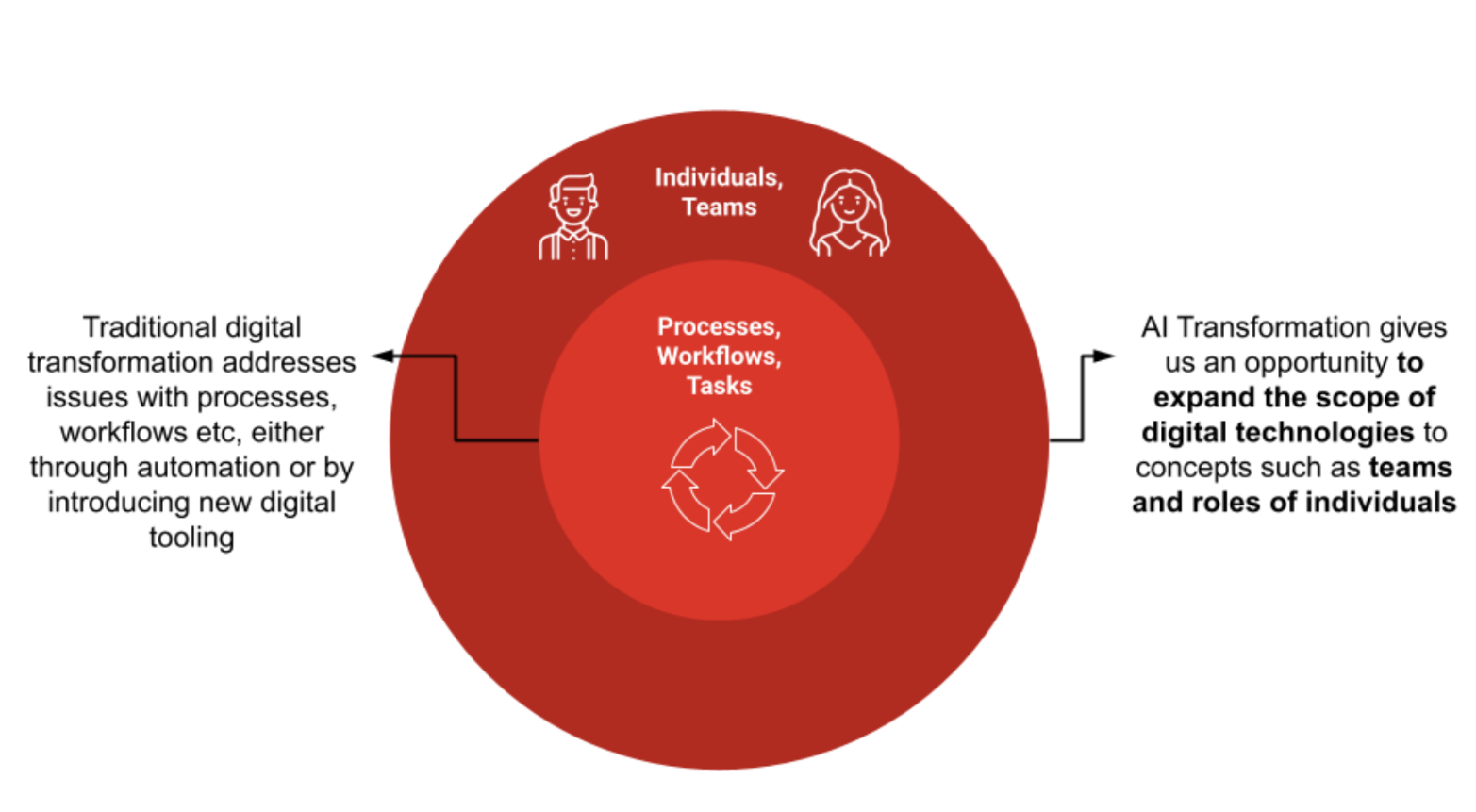
To do so, we need to abandon the old ways of thinking about digital technologies and apply a new set of strategies to integrate AI into our organizations. These strategies have to elevate technology to the level of the human and answer a host of new questions, from organisational and governance questions, to fairness and sustainability. We call this: the AI transformation.
The Monolithic AI Bet
Recent years have seen a strong push and enormous investments in developments of ever-larger AI models, with the ultimate goal of developing Artificial General Intelligence. In other words, AI models that can do almost everything. This is what large tech spearheaded by OpenAI, Anthropic etc. have been trying to achieve. To give you a sense of the strength of their commitment: OpenAI, Oracle and Softbank announced a 500 billion dollar investment in AI infrastructure. This is a true testament to the resources companies and individuals are willing to invest into development of monolithic AI.
“One model for everything” is an attractive idea. It is convenient and easily commoditised, but it also comes with a large baggage of issues:
- Monolithic AI is power hungry. It takes an enormous amount of resources, from electricity to raw materials to develop and power it, raising serious questions about the sustainability of this approach, even in the near term.
- The models are so large (in memory and computing needs) that they can only be hosted in large data centers. This means that as a user, you have to be comfortable with the fact that your data will, at some point, have to go to the cloud.
- Monolithic AI is developed and controlled by a handful of large tech companies. This means that you have to be comfortable to outsource not only your data, but your intelligence to a third party.
- No one really knows or fully understands how these models work or why. They are close-sourced and you have nothing but trust in large tech companies to rely on when it comes to explaiability and accountability of the model predictions
- If one model is doing everything, what happens when it fails? Resilience is a real issue you have no control over with monolithic AI models.
These issues have so far been mostly shoved under the rug by companies which develop AI, but they are central for most companies that want to work with AI!DeepSeek, an AI model developed using mere 5 million dollars of resources and capabilities on par with state of the art GPT models of OpenAI, which took hundreds of millions of dollars to develop, caused a shock in the markets yesterday. A trillion dollars in market value went up in smoke in one day. NVIDIA suffered the highest stock price drop in the history of the stock market. Why did this happen? People realised that bigger is not necessarily better, especially if smaller models can help you address some of the abovementioned issues. The story that investments on par with the moon-landing are the only way to improve AI turned out not to be true.
The right way to conduct AI Transformation
If the old ways won’t work for AI transformation, what will? AI is not just another digital tool, it is an assistant. We have to think of AI not at the level of “how will humans use AI”, but “how will humans work with AI?” In addition, AI is a transversal technology, able to affect every aspect of a business, from core operations to business strategy. All of this opens a host of new questions which we have to answer:
- Where in my organisation should AI play a role?
- How will AI affect the role of humans in an organisation?
- Should AI automate all it can?
- Which AI model should I use, and for what?
- What is the impact of introducing AI on sustainability and resilience of my organisation?
To address these and other questions, we have developed a new AI transformation value proposition.
1. Holistic perspective over narrow goals: Correct AI strategy emphasizes change management, strategic planning, and cultural alignment to ensure AI becomes a transformative part of business operations. By engaging stakeholders at all levels, it fosters collaboration, addresses unique challenges, and unlocks the full potential of AI for meaningful change.
2. Human empowerment over machine autonomy: Many AI projects fail because they mistakenly aim to replace humans entirely, leading to unmet expectations and frustration. We focus on creating AI systems that empower and elevate humans, acting as collaborative partners with imperfections and room for improvement. By prioritizing thoughtful human experience design, we ensure these systems enhance human abilities while maintaining a sense of control and trust.
3. Collaborative intelligence over monolithic intelligence: Recent AI advancements may suggest that monolithic, all-knowing systems are achievable with vast computing power and large datasets, but this view is misleading. True intelligence arises from collaboration among multiple agents and their ability to engage transparently with humans, not merely from the size of a single model. Embracing "multi-agent systems", we focus on creating sustainable AI systems that leverage collaborative intelligence to overcome traditional limitations.
4. Sovereignty over convenience: Cloud computing has transformed IT by decentralizing resources and enabling scalable AI models offered "as-a-service," but this often involves sharing sensitive data and facing unpredictable costs and updates. We advocate for critically assessing proprietary AI cloud services, especially beyond the early stages of AI transformation. Prioritizing infrastructures that provide greater control over data privacy and the AI itself ensures financial efficiency and long-term sovereignty. Just as your data should belong to you, so should your intelligence.
5. Sustainability and resilience over short term performance: We envision AI transformation as sustainable and resilient, prioritizing long-term growth and positive impact over short-term gains. By integrating green computing techniques and adhering to high ethical standards, we create AI solutions that harmonize with ecological and social environments. Robust and adaptable designs should ensure that AI transformation is both sustainable and resilient. This is why we advocate for focusing on open architectures, ensuring safety and flexibility in navigating evolving technologies, regulations, and market dynamics.
Are you convinced? Great! The only question that remains is: how do we turn these ideas into reality? The answer is: multi-agent systems.
Multi-agent systems are the future of AI
You’ve probably heard a lot about agentic AI lately, and if you’ve ever used ChatGPT or Microsoft Copilot, you’ve interacted with an AI agent. To put it simply, a common definition of an AI agent is an AI model which can use “tools” (e.g. search engine, access to a database, documents, calculators etc.). I find this definition a bit too constricting. To me, an agent is anything that can perform intellectual operations, use tools and exchange information.
So far, much effort has been dedicated to building single agents which are meant to interact with humans in a one-to-one manner. It is a natural step forward from large language models. But is it the only way to interact with AI?
The next step in this evolution is to consider many AI and human agents working together as a team. This is a substantial challenge as we have to answer questions such as who should talk to whom and when, how should the labour be divided amongst the team and who should be responsible for which outcome. This, by the way, is exactly what we do with human-only organisations.
Multi-agents also open up so many new opportunities to use AI. Consider a typical setup of Microsoft Copilot. It functions as an assistant to a single human, who can use it to access data, analyse it etc. There are several important features of the setup:
- Data processing is performed by a monolithic AI model (e.g. GPT-4) in the cloud
- The setup is envisioned to be generalist. The only way to “fine tune” model performance is by prompt engineering
- You can impose data governance, e.g. via role based access, but at some point your data must leave your physical data storage to be analysed by the model in the cloud.
- Two Copilots can not talk to each other (the setup is meant for 1-1 interaction)
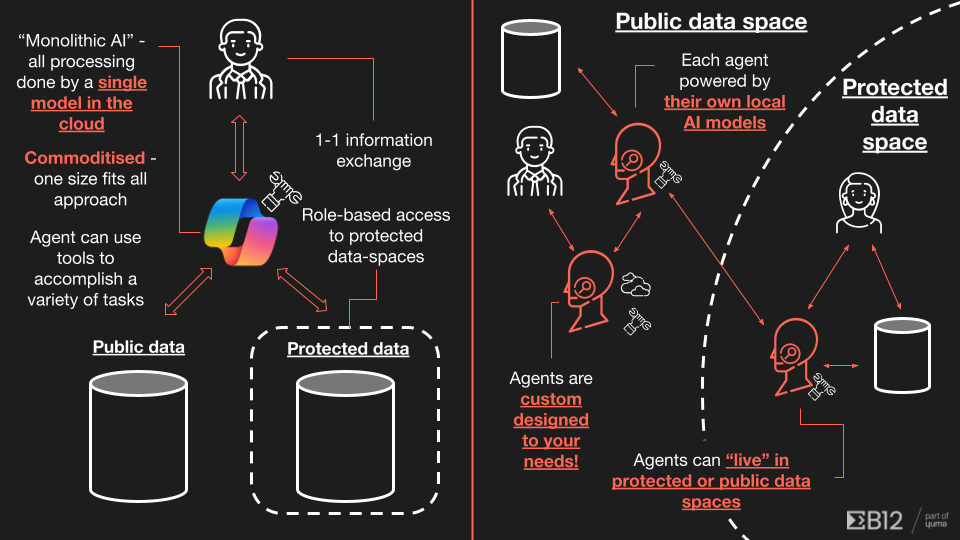
This is hardly sufficient in many business settings. Nowadays we can design systems where many agents and humans work in a team, together. Agents can exchange information between each other and physically “live” in protected dataspaces so your data never has to leave your infrastructure. Each agent can be powered by a different AI model, or by deterministic code. They can interact with humans in similar ways to Copilot and ChatGPT, but also have unique personalities, roles and skills which we get to define. They can ask questions and verifications from humans when needed to ensure that you have accountability over the outcomes. Multi-agent systems allow you to identify where humans can excel, and even elevate their roles into, say, “managers of AI agents”. Such systems could be the next revolution in AI!
Multi-agent systems should not be mistaken for steps in a workflow or a process, but truly be seen as a hybrid human-AI team. As such, they support concepts such as structure, process, workflow etc, but don’t insist on it. This flexibility of multi-agent paradigm is one of the greatest opportunity generators!
Conclusions
The transformative potential of AI lies in its ability to work with humans rather than simply being another tool for them to use. The era of rigid processes and workflows is giving way to flexible, team-based collaboration between human and AI agents, a shift that demands a fundamental rethinking of how we approach digital transformation. Multi-agent systems embody this evolution, enabling seamless, adaptable teamwork while addressing the challenges of scalability, governance, and sustainability. By embracing a holistic, human-centered approach to AI transformation, we can unlock unprecedented levels of productivity and innovation. The key to success lies in adopting strategies that elevate technology to the level of the human, fostering collaboration, and creating systems that are not only intelligent but resilient, sustainable, and empowering. The future of AI isn’t about tools—it’s about teams.
References
- Gartner report (2024-05-07): https://www.gartner.com/en/newsroom/press-releases/2024-05-07-gartner-survey-finds-generative-ai-is-now-the-most-frequently-deployed-ai-solution-in-organizations
About the author
Mihailo Backović started as a theoretical physicist turned AI expert who specializes in guiding organizations through successful AI transformations. As a Managing Partner at B12 Consulting | part of Yuma, he is helping numerous companies—from biotech and healthcare to mobility and finance—harness AI to achieve their biggest ambitions. Known for his deep analytical approach and passion for tackling complex problems, Mihailo is dedicated to shaping a future where AI and humanity thrive together. He actively explores the transformative impact of AI on the topics such as the job market, democracy, disinformation, and is committed to building AI solutions that empower people and businesses to flourish in an increasingly automated world.